Collaborative Research: CNS Core: Medium: Towards Federated Learning over 5G Mobile Devices: High Efficiency, Low Latency, and Good Privacy
Project Information
- Collaborative Research: CNS Core: Medium: Towards Federated Learning over 5G Mobile Devices: High Efficiency, Low Latency, and Good Privacy, National Science Foundation, October 1, 2021 – September 30, 2025. This is a collaborative project of University of Houston (PI: Dr. Miao Pan, Co-PI: Dr. Xin Fu), University of Florida (PI: Dr. Yuguang Fang 21-22, Dr. Tan Wong 22-Now), and University of Texas at San Antonio (PI: Dr. Yanming Gong, Co-PI: Dr. Yuanxiong Guo). University of Houston is the leading institution.
- This webpage and the materials provided here are based upon work supported by the National Science Foundation.
Project Synopsis
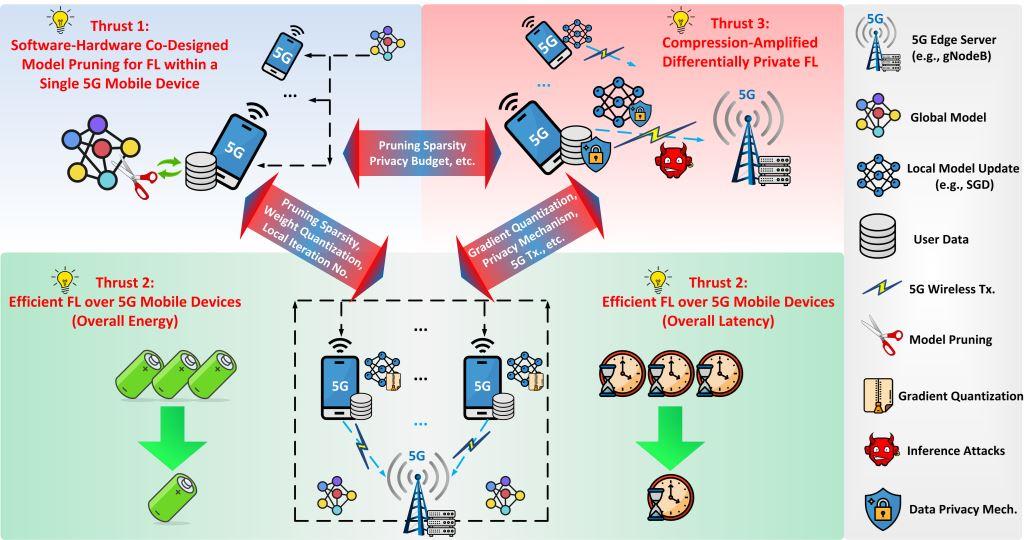
Recent emerging federated learning (FL) allows distributed data sources to collaboratively train a global model without sharing their privacy sensitive raw data. However, due to the huge size of the deep learning model, the model downloads and updates generate significant amount of network traffic which exerts tremendous burden to existing telecommunication infrastructure. This project takes FL over 5G mobile devices as a workable application scenario to address this dilemma, which will significantly improve the design, analysis and implementation of FL over 5G mobile devices. The research outcomes will substantially enrich the knowledge of machine learning technologies and 5G systems and beyond. Moreover, this project is multidisciplinary, involving machine learning/deep learning/federated learning, edge computing, wireless communications and networking, security and privacy, computer architectural design, etc., which will serve as a fruitful training ground for both graduate and undergraduate students to equip them with multidisciplinary skills for future work force to boost the national economy. Furthermore, outreach activities to high school students will increase the participation of female and minority students in science and engineering.
Specifically, by observing that iterative model updates tend to show high sparsity, the investigators leverage model update sparsity to design model pruning and quantization schemes to optimize local training and privacy-preserving model updating in order to lower both energy consumption and model update traffic. They achieve this design goal by conducting the four research tasks: (1) designing software-hardware co-designed model pruning schemes and adaptive quantization techniques in FL within a single 5G mobile device according to the local data and model sparsity property to reduce the local computation and memory access; (2) making sound trade-off between “working” (i.e., local computing) and “talking” (i.e., 5G wireless transmissions) to boost the overall energy/communications efficiency for FL over 5G mobile devices; (3) developing novel differentially private compression schemes based on sparsification property and quantization adaptability to rigorously protect data privacy while maintaining high model accuracy and communication efficiency in FL; and (4) building a testbed to thoroughly evaluate the proposed designs.
Personnel and Collaborators
Personnel at UH
- Dr. Miao Pan, PI
- Dr. Xin Fu, Co-PI
- Ms. Rui Chen, PhD (Graduated in Summer, 2023)
- Mr. Dian Shi, PhD (Graduated in Summer, 2022)
- Ms. Pavana Prakash, PhD (Graduated in Fall, 2023)
- Mr. Qiyu Wan, PhD (Graduated in Fall, 2022)
- Mr. Lening Wang, PhD Candidate
- Mr. Huai-an Su, PhD Student
Personnel at UF
- Dr. Yuguang Fang, PI (2021-2022)
- Dr. Tan Wong, PI (2022-Present)
- Mr. Guangyu Zhu, PhD Student
Personnel at UTSA
- Dr. Yanmin Gong, PI
- Dr. Yuanxiong Guo, Co-PI
- Mr. Zhidong Gao, PhD Student
- Mr. Zhenxiao Zhang, PhD Student
Research Progress and Outreach Activities
Publications
- Rui Chen, Qiyu Wan, Pavana Prakash, Lan Zhang, Xu Yuan, Yanmin Gong, Xin Fu and Miao Pan, “Workie-Talkie: Accelerating Federated Learning by Overlapping Computing and Communications via Contrastive Regularization” IEEE/CVF International Conference on Computer Vision 2023 (ICCV).
- Peichun Li, Guoliang Cheng, Xumin Huang, Jiawen Kang, Rong Yu, Yuan Wu, and Miao Pan, “AnycostFL: Efficient On-Demand Federated Learning over Heterogeneous Edge Devices”, IEEE International Conference on Computer Communications 2023 (INFOCOM).
- Prakash, Pavana and Ding, Jiahao and Chen, Rui and Qin, Xiaoqi and Shu, Minglei and Cui, Qimei and Guo, Yuanxiong and Pan, Miao. (2022). IoT Device Friendly and Communication-Efficient Federated Learning via Joint Model Pruning and Quantization. IEEE Internet of Things Journal.
- Chen, Rui and Wan, Qiyu and Zhang, Xinyue and Qin, Xiaoqi and Hou, Yanzhao and Wang, Di and Fu, Xin and Pan, Miao. (2023). EEFL: High-Speed Wireless Communications Inspired Energy Efficient Federated Learning over Mobile Devices. ACM International Conference on Mobile Systems, Applications, and Services (MobiSys).
- Chen, Rui and Shi, Dian and Qin, Xiaoqi and Liu, Dongjie and Pan, Miao and Cui, Shuguang. (2023). Service Delay Minimization for Federated Learning Over Mobile Devices. IEEE Journal on Selected Areas in Communications.
- Zhang, Xinyue and Chen, Rui and Wang, Jingyi and Zhang, Huaqing and Pan, Miao. (2022). Energy Efficient Federated Learning over Cooperative Relay-Assisted Wireless Networks. IEEE Global Communications Conference.
- Shi, Dian and Li, Liang and Wu, Maoqiang and Shu, Minglei and Yu, Rong and Pan, Miao and Han, Zhu. (2022). To Talk or to Work: Dynamic Batch Sizes Assisted Time Efficient Federated Learning Over Future Mobile Edge Devices. IEEE Transactions on Wireless Communications.
- Wang, Lening and Sistla, Manojna and Chen, Mingsong and Fu, Xin. (2022). BS-pFL: Enabling Low-Cost Personalized Federated Learning by Exploring Weight Gradient Sparsity. International Joint Conference on Neural Networks (IJCNN).
- Chen, Rui and Li, Liang and Xue, Kaiping and Zhang, Chi and Pan, Miao and Fang, Yuguang. (2022). Energy Efficient Federated Learning over Heterogeneous Mobile Devices via Joint Design of Weight Quantization and Wireless Transmission. IEEE Transactions on Mobile Computing.
- Shi, Dian and Li, Liang and Chen, Rui and Prakash, Pavana and Pan, Miao and Fang, Yuguang. (2022). Towards Energy Efficient Federated Learning over 5G+ Mobile Devices. IEEE Wireless Communications.
- Prakash, Pavana and Ding, Jiahao and Wu, Maoqiang and Shu, Minglei and Yu, Rong and Pan, Miao. (2021). To Talk or to Work: Delay Efficient Federated Learning over Mobile Edge Devices. IEEE Global Communications Conference 2021.
- Prakash, Pavana and Ding, Jiahao and Chen, Rui and Qin, Xiaoqi and Shu, Minglei and Cui, Qimei and Guo, Yuanxiong and Pan, Miao. (2022). IoT Device Friendly and Communication-Efficient Federated Learning via Joint Model Pruning and Quantization. IEEE Internet of Things Journal.
- Wan, Qiyu and Xia, Haojun and Zhang, Xingyao and Wang, Lening and Song, Shuaiwen Leon and Fu, Xin. (2021). Shift-BNN: HighlyEfficient Probabilistic Bayesian Neural Network Training via Memory-Friendly Pattern Retrieving. IEEE/ACM International Symposium on Microarchitecture (MICRO).
- Zhang, Zhenxiao and Guo, Yuanxiong and Fang, Yuguang and Gong, Yanmin (2023). Communication and Energy Efficient Wireless Federated Learning With Intrinsic Privacy. IEEE Transactions on Dependable and Secure Computing.
- Wei, Xinliang and Fan, Lei and Guo, Yuanxiong and Gong, Yanmin and Han, Zhu and Wang, Yu (2024). Hybrid Quantum-Classical Benders’ Decomposition for Federated Learning Scheduling in Distributed Networks. IEEE Transactions on Network Science and Engineering.
- Zhang, Yu and Gong, Yanmin and Guo, Yuanxiong (2024). Semi-Supervised Federated Learning for Assessing Building Damage from Satellite Imagery, IEEE ICC 2024.
- Wei, Xinliang and Fan, Lei and Guo, Yuanxiong and Gong, Yanmin and Han, Zhu and Wang, Yu (2024). Hybrid Quantum-Classical Benders’ Decomposition for Federated Learning Scheduling in Distributed Networks. IEEE Transactions on Network Science and Engineering.
- Hu, Rui and Guo, Yuanxiong and Gong, Yanmin (2024). Federated Learning With Sparsified Model Perturbation: Improving Accuracy Under Client-Level Differential Privacy. 23. (8). IEEE Transactions on Mobile Computing.
- Gao, Zhidong and Guo, Yuanxiong and Gong, Yanmin (2023). One Node Per User: Node-Level Federated Learning for Graph Neural Networks. NeurIPS Workshop on New Frontiers in Graph Learning.
- Jeffrey Jiarui Chen, Rui Chen, Xinyue Zhang, and Miao Pan, “A Privacy Preserving Federated Learning Framework for COVID-19 Vulnerability Map Construction”, IEEE International Conference on Communications (ICC’21), Virtual/Montreal, Canada, June 14-23, 2021.
- Rui Chen, Liang Li, Jeffrey Jiarui Chen, Ronghui Hou, Yanmin Gong, Yuanxiong Guo, and Miao Pan, “COVID-19 Vulnerability Map Construction via Location Privacy Preserving Mobile Crowdsourcing“, IEEE Global Communications Conference (GLOBECOM’20), Taipei, Taiwan, December 7-11, 2020.
- Hao Gao, Wuchen Li, Miao Pan, Zhu Han, and H. Vincent Poor, “Analyzing Social Distancing and Seasonality of COVID-19 with Mean Field Evolutionary Dynamics”, IEEE Global Communications Conference (GLOBECOM’20) Special Workshop on Communications and Networking Technologies for Responding to COVID-19, Taipei, Taiwan, December 7-11, 2020.
Links to Code Repositories
- Github Code Links: https://github.com/RuiC8/MapApp